Answer:
a. The Poisson approximation is good because n is large, p is small, and np < 10.
The parameter of thr Poisson distribution is:

b. P(r=0)=0.2019
c. P(r>1)=0.4751
d. P(r>2)=0.2167
e. P(r>3)=0.0789
Explanation:
a. The Poisson distribution is appropiate to represent binomial events with low probability and many repetitions (small p and large n).
The approximation that the Poisson distribution does to the real model is adequate if the product np is equal or lower than 10.
In this case, n=963 and p=1/596, so we have:

The Poisson approximation is good because n is large, p is small, and np < 10.
The parameter of thr Poisson distribution is:

We can calculate the probability for k events as:
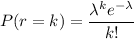
b. P(r=0). We use the formula above with λ=1.6 and r=0.

c. P(r>1). In this case, is simpler to calculate the complementary probability to P(r<=1), that is the sum of P(r=0) and P(r=1).
![P(r>1)=1-P(r\leq1)=1-[P(r=0)+P(r=1)]\\\\\\P(0)=1.6^(0) \cdot e^(-1.6)/0!=1*0.2019/1=0.2019\\\\P(1)=1.6^(1) \cdot e^(-1.6)/1!=1.6*0.2019/1=0.3230\\\\\\P(r>1)=1-(0.2019+0.3230)=1-0.5249=0.4751](https://img.qammunity.org/2021/formulas/mathematics/college/s5jeka74xjh61ezraac9ukvuo5edg3x79l.png)
d. P(r>2)
![P(r>2)=1-P(r\leq2)=1-[P(r=0)+P(r=1)+P(r=2)]\\\\\\P(0)=1.6^(0) \cdot e^(-1.6)/0!=1*0.2019/1=0.2019\\\\P(1)=1.6^(1) \cdot e^(-1.6)/1!=1.6*0.2019/1=0.3230\\\\P(2)=1.6^(2) \cdot e^(-1.6)/2!=2.56*0.2019/2=0.2584\\\\\\P(r>2)=1-(0.2019+0.3230+0.2584)=1-0.7833=0.2167](https://img.qammunity.org/2021/formulas/mathematics/college/sx1hnprh5wohd0tsfpwzamfydagzcbwesu.png)
e. P(r>3)
![P(r>3)=1-P(r\leq2)=1-[P(r=0)+P(r=1)+P(r=2)+P(r=3)]\\\\\\P(0)=1.6^(0) \cdot e^(-1.6)/0!=1*0.2019/1=0.2019\\\\P(1)=1.6^(1) \cdot e^(-1.6)/1!=1.6*0.2019/1=0.3230\\\\P(2)=1.6^(2) \cdot e^(-1.6)/2!=2.56*0.2019/2=0.2584\\\\P(3)=1.6^(3) \cdot e^(-1.6)/3!=4.096*0.2019/6=0.1378\\\\\\P(r>3)=1-(0.2019+0.3230+0.2584+0.1378)=1-0.9211=0.0789](https://img.qammunity.org/2021/formulas/mathematics/college/4qwx4llafxfuzq1z4wkui5wa9vjxlrpkg0.png)