Answer:
(i) The estimated regression equation is;
≈ 1.6896 + 0.0604·X
The coefficient of 'X' indicates that
increase by a multiple of 0.0604 for each million dollar increase in sales, X
(ii) The estimated earnings for the company is approximately $4.7096 million
(iii) The standard error of estimate is approximately 29.34
The high standard error of estimate indicates that individual mean do not accurately represent the population mean
(iv) The coefficient of determination is approximately 0.57925
The coefficient of determination indicates that the probability of the coordinate of a new point of data to be located on the line is 0.57925
Explanation:
The given data is presented as follows;
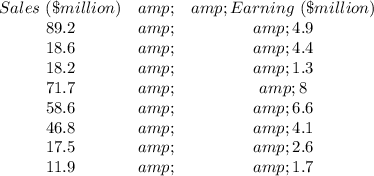
(i) From the data, we have;
The regression equation can be presented as follows;
= b₀ + b₁·x
Where;
b₁ = The slope given as follows;
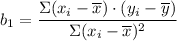
b₀ =
- b₁·

From the data, we have;
= 364.05
= 6,027.259
= 4.2
= 41.5625
∴ b₁ = 364.05/6,027.259 ≈ 0.06040059005
b₀ = 4.2 - 0.06040059005 × 41.5625 ≈ 1.68960047605 ≈ 1.69
Therefore, we have the regression equation as follows;
≈ 1.6896 + 0.0604·X
The coefficient of 'X' indicates that the earnings increase by a multiple of 0.0604 for each million dollar increase in sales
(ii) For the small company, we have;
X = $50.0 million, therefore, we get;
= 1.6896 + 0.0604 × 50 = 4.7096
The estimated earnings for the company,
= 4.7096 million
(iii) The standard error of estimate, σ, is given by the following formula;
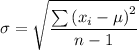
Where;
n = The sample size
Therefore, we have;
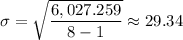
The standard error of estimate, σ ≈ 29.34
The high standard error of estimate indicates that it is very unlikely that a given mean value within the data is a representation of the true population mean
(iv) The coefficient of determination (R Square) is given as follows;

Where;
SSR = The Sum of Squared Regression ≈ 21.9884
SST = The total variation in the sample ≈ 37.96
Therefore, R² ≈ 21.9884/37.96 ≈ 0.57925
The coefficient of determination, R² ≈ 0.57925.
Therefore, by the coefficient of determination, the likelihood of a new introduced data point to located on the line is 0.57925