Answer:
The probability that the sample mean is more than 110 is 0.0384.
Explanation:
According to the Central Limit Theorem if we have an unknown population with mean μ and standard deviation σ and appropriately huge random samples (n > 30) are selected from the population with replacement, then the sampling distribution of the sample mean will be approximately normally distributed.
Then, the mean of the sampling distribution of sample mean is given by:

And the variance of the sampling distribution of sample mean is given by:

The information provided is:

Since n = 50 > 30, the central limit theorem can be applied to approximate the sampling distribution of sample mean by the normal distribution.
The mean variance of the sampling distribution for the sample mean are:
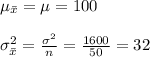
That is,
.
Compute the probability that the sample mean is more than 110 as follows:

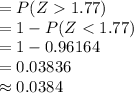
*Use a z-table.
Thus, the probability that the sample mean is more than 110 is 0.0384.