Answer:
Explanation:
Hello!
You have to calculate the linear correlation coefficient (r) between the variables
X₁: temperature
X₂: Number of ice cream cones sold per hour.
The formula to calculate the coefficient of linear correlation between the two variables is the following:
![r= \frac{sumX_1X_2-((sumX_1)(sumX_2))/(n) }{\sqrt{[sumX_1^2-((sumX_1)^2)/(n) ][sumX_2^2((sumX_2)^2)/(n) ]} }](https://img.qammunity.org/2021/formulas/mathematics/college/dvymcsnzsr561f90keqheul5rbqlufb2xi.png)
∑X₁= 765; ∑X₁²= 66525 ∑X₂= 134 ∑X₂²= 2228 ∑X₁X₂= 11965 n=9
![r= \frac{11965-(765*134)/(9) }{\sqrt{[66525-((765)^2)/(9) ][2228-((134)^2)/(9) ]} }](https://img.qammunity.org/2021/formulas/mathematics/college/cxfwg5tvd2lyazwqqw59ewqdmk912n1vnp.png)
r= 0.97
To test if there is a positive linear association between both variables the statistical hypotheses are:
H₀: ρ ≤ 0
H₁: ρ > 0
critical value 0.666
r= 0.97
The decision is to reject the null hypothesis, there is a positive linear correlation between the temperature and the number of ice cream cones sold per hour.
The regression line is
^Y= a + bX
where
a: represents the estimation of the y-intercept
b: represents the estimation of the slope
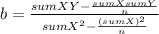
b= 0.38
a= Y[bar]-bX[bar]
a= -17.69
The estimated regression line for the number of ice cream cones and the temperature is ^Y= -17.69 + 0.38Xi
To estimate the number of ice cream cones sold when the temperature is 80º you have to replace the value of X in the estimated regression line:
^Y= -17.69 + 0.38*80= 12.71
It is expected that they will sell 12.71 ≅ 13 ice cream cones when the temperature is 80º
You can use the estimation of the regression line to predict values within the range of definition of the independent variable, in this case from 65º to 105º of temperature, because it is within those values that you can be sure they have a linear association. Below or above you are not certain that their association is linear, it may be, for example exponential, or there may be no association between them.
Example: If the temperature is comfortable or cold, it is more likely that people won't buy ice creams, so for those values it will be no linear association between them.
I hope this helps!