Answer:
a) P(550 < x < 1300) = 0.9759
b) P(900 < x < 1100) = 0.9652
P(850 < x < 1300) = 0.9992
Explanation:
To solve this question, we need to understand the normal probability distribution and the central limit theorem.
Normal probability distribution
Problems of normally distributed samples are solved using the z-score formula.
In a set with mean
and standard deviation
, the zscore of a measure X is given by:

The Z-score measures how many standard deviations the measure is from the mean. After finding the Z-score, we look at the z-score table and find the p-value associated with this z-score. This p-value is the probability that the value of the measure is smaller than X, that is, the percentile of X. Subtracting 1 by the pvalue, we get the probability that the value of the measure is greater than X.
Central Limit Theorem
The Central Limit Theorem estabilishes that, for a normally distributed random variable X, with mean
and standard deviation
, the sampling distribution of the sample means with size n can be approximated to a normal distribution with mean
and standard deviation
.
For a skewed variable, the Central Limit Theorem can also be applied, as long as n is at least 30.
In this problem, we have that:

a) P(550 < x < 1300) =
pvalue of Z when X = 1300 subtracted by the pvalue of Z when X = 550.
X = 1300

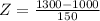

has a pvalue of 0.9772
X = 550



has a pvalue of 0.0013
0.9772 - 0.0013 = 0.9759
P(550 < x < 1300) = 0.9759
b)
Now we have

P(900 < x < 1100) =
pvalue of Z when X = 1100 subtracted by the pvalue of Z when X = 900.
X = 1100

By the Central Limit Theorem

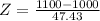

has a pvalue of 0.9826
X = 900



has a pvalue of 0.0174
0.9826 - 0.0174 = 0.9652
P(900 < x < 1100) = 0.9652
P(850 < x < 1300) =
pvalue of Z when X = 1300 subtracted by the pvalue of Z when X = 850. So
X = 1300

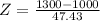

has a pvalue of 1
X = 850



has a pvalue of 0.0008
1 - 0.0008 = 0.9992
P(850 < x < 1300) = 0.9992