Answer:
a)
For this case the value for
is always smaller than the value of a, assuming
So then for this case it cannot be unbiased because an unbiased estimator satisfy this property:
and that's not our case.
b)

Since is a negative value we can conclude that underestimate the real value a.

c)

And assuming independence we have this:
![P(Y \leq y) = P(X_1 \leq y) P(X_2 \leq y) .... P(X_n \leq y) = [P(X_1 \leq y)]^n = ((y)/(a))^n](https://img.qammunity.org/2021/formulas/mathematics/college/jbu04wm4yz07vffaj1p1gd791h5t7yhxow.png)
![f_Y (Y) = n ((y)/(a))^(n-1) * (1)/(a)= (n)/(a^n) y^(n-1) , y \in [0,a]](https://img.qammunity.org/2021/formulas/mathematics/college/3kgg0m3r2pn4n6dzr48lbs7dl6rnldzi8b.png)
e) On this case we see that the estimator
is better than
and the reason why is because:


and that's satisfied for n>1.
Explanation:
Part a
For this case we are assuming
And we are are ssuming the following estimator:
For this case the value for
is always smaller than the value of a, assuming
So then for this case it cannot be unbiased because an unbiased estimator satisfy this property:
and that's not our case.
Part b
For this case we assume that the estimator is given by:

And using the definition of bias we have this:

Since is a negative value we can conclude that underestimate the real value a.
And when we take the limit when n tend to infinity we got that the bias tend to 0.

Part c
For this case we the followng random variable
and we can find the cumulative distribution function like this:

And assuming independence we have this:
![P(Y \leq y) = P(X_1 \leq y) P(X_2 \leq y) .... P(X_n \leq y) = [P(X_1 \leq y)]^n = ((y)/(a))^n](https://img.qammunity.org/2021/formulas/mathematics/college/jbu04wm4yz07vffaj1p1gd791h5t7yhxow.png)
Since all the random variables have the same distribution.
Now we can find the density function derivating the distribution function like this:
![f_Y (Y) = n ((y)/(a))^(n-1) * (1)/(a)= (n)/(a^n) y^(n-1) , y \in [0,a]](https://img.qammunity.org/2021/formulas/mathematics/college/3kgg0m3r2pn4n6dzr48lbs7dl6rnldzi8b.png)
Now we can find the expected value for the random variable Y and we got this:

And the bias is given by:

And again since the bias is not 0 we have a biased estimator.
Part e
For this case we have two estimators with the following variances:

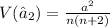
On this case we see that the estimator
is better than
and the reason why is because:


and that's satisfied for n>1.