We have that 20% of the women biopsed have breast cancer. If the sample size is 200,000, then we have that:

then, there are 40,000 women with breast cancer.
Next, we have that the test is positive for approximately 2% of the women that don't have breast cancer. Then, if the remaining 160,000 women of the sample don't have breast cancer, we have that:

we also have that the test is negative for the 5% that do have breast cancer, then we have:

With this information we can fill the hypothetical frequence table:
Finally, to calculate the probability that a woman does not have breast cancer given that the test is negative, we can use conditional probability to find out:
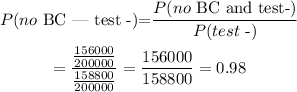
therefore , the probability is 0.98 = 98%