(a) In this question, we can focus on the columns for Age and Height only.
Looking into it, we can see that, we have some players with similar ages of 24, 25, 26 that have totally different heights, 193, 173, 183 and 185.
We also can see that some players with same height of 185 have very different ages, like 26, 35, 27, 28.
This means that it doesn't appear to be a relationship between age and height in this case.
So, the the best answer is the last on: "No, it does not seems likely that there is a strong association."
(b) Using a calculation tool, we can calculate the correlation between the columns "Age" and "Height". The others are not used.
The correlation coefficient obtained when we do that is approximately 0.1207.
(c) Since the correlation coefficient is positive, the first dropdown is "positive".
Since the correlation coefficient is close to 0 (not close to 1 or -1), the answer weak or negligible. Since it is very close to zero, it probably is negligible, but this classification is relative.
(d) Similar to hat we have done for the correlation coefficient, we can use a calculation tool to get the regression for theses columns. Since we want to predict the "Height" using the "Age", we have to use "Age" as the independent varible, "x", and "Height" as the dependent variable, "y".
Doing this, we get the regression equation:

(e) With the regression equation, we can predict the height of a player with age 26 by substituting 26 into "x":
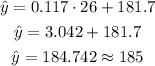
So, the prediction of the equation is 185 cm.