Part (a)
Given data table.

where,
- t = time in minutes
- T = temperature in Celsius
It's unfortunate that T is used twice for these variables. It might cause some confusion.
This is what happens when we subtract 24 from each temperature.
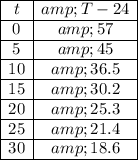
The graph of each set of points is shown in figure A. I used GeoGebra to make the graph.
The first set of data points are in red. The second set in blue. Notice the set of blue points is the result of shifting the red points 24 units down.
The green exponential model is also shown as the curve through the set of red points.
The green regression exponential model doesn't go through each point perfectly. Rather it tries to get as close as possible.
To go from
T-24 = 54.4(0.964)^t
to
T = 54.4(0.964)^t + 24
we simply add 24 to both sides. This means there isn't much to show in terms of steps when solving for T.
=======================================================
Part (b)
Use spreadsheet technology or something like GeoGebra to generate this data table.
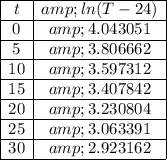
The values in the 2nd column are approximate.
Then plot each of those points on the same xy grid. Use technology of your choice to determine the regression line is roughly
y = -0.03723x + 3.99739
So we can say
ln(T-24) = -0.03723t + 3.99739
the right hand side fits the format at + b where
both of which are approximate.
Then,
e^(ln(T-24)) = e^(at+b)
T-24 = e^(at+b)
T-24 = e^(-0.03723t + 3.99739)
T-24 = e^(-0.03723t)*e^(3.99739)
T-24 = e^(-0.03723t)*54.45583
T-24 = 54.45583*e^(-0.03723t)
T = 54.45583*e^(-0.03723t) + 24
T = 54.456*e^(-0.037t) + 24
Let's verify that this is equivalent to the model found in part (a).
T = 54.456*e^(-0.037t) + 24
T = 54.456*(e^(-0.037))^t + 24
T = 54.456*(0.963676)^t + 24
T = 54.5*(0.964)^t + 24
which is very close to the model mentioned in part (a). There's some slight rounding error. That's to be expected in problems like this.
-----------------------------
Answer: T = 54.456*e^(-0.037t) + 24
See figure B for the graph. Like with the other regression curve, this straight line doesn't go through all of the points. It tries to get as close as possible.
=======================================================
Part (c)
Refer to the 2nd table mentioned in part (a).
We'll apply the reciprocal to each item in the column labeled T-24.
For instance, the item 57 becomes 1/57 = 0.017544 approximately.
This is what the data table looks like:
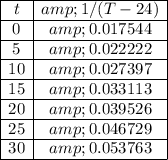
The values in the 2nd column are approximate.
The set of points and regression line are shown in figure C1. This line does not go through all of the points. Like with the others, it tries to get as close as possible to each point.
Use technology to determine the equation of the regression line is approximately:
y = 0.00121x + 0.01613
Which tells us that
1/(T-24) = 0.00121t + 0.01613
Let's solve for uppercase T.
1/(T-24) = 0.00121t + 0.01613
T-24 = 1/(0.00121t + 0.01613)
T = 1/(0.00121t + 0.01613) + 24
T = 1/(0.0012t + 0.0161) + 24
which is the approximate final answer.
Figure C2 shown below represents the original data points (t, T) in red. The blue curve is y = 1/(0.0012x+0.0161)+24, which is the rational regression curve we just found. This curve tries its best to get as close as possible to each red point.
Compare the regression curves of figure A and figure C2. We have a very close tie in terms of which curve does a better job of fitting the original data.